In today’s data-driven world, businesses are constantly bombarded with vast amounts of information. But how can they harness the power of this data to drive success? Enter data analysis. By leveraging the right tools and techniques, businesses can transform data into actionable insights that can guide strategic decisions and optimize processes.
In this article, we delve into the world of data analysis and explore how it can unlock the secrets hidden within the numbers. From identifying trends and patterns to making data-driven predictions, data analysis offers a powerful toolset for businesses in their quest for success.
Whether you’re a small startup or a multinational corporation, data analysis can provide you with a competitive edge by enabling you to make informed decisions, improve efficiency, and enhance customer satisfaction. Join us as we dive into the fascinating world of data analysis and discover how it can help your business thrive in today’s fast-paced digital landscape.
So, buckle up and get ready to unlock the power of data analysis. The insights you uncover may just pave the way for your business’s future success.
Why Data Analysis is Important for Businesses?
In the rapidly evolving landscape of modern business, data analysis has emerged as a cornerstone for informed decision-making. Organizations are inundated with data from various sources—sales figures, customer feedback, social media interactions, and market trends, to name a few.
Identifying Patterns & Correlations
The ability to sift through this ocean of information and extract meaningful insights can mean the difference between thriving and merely surviving. Data analysis enables businesses to identify patterns and correlations, leading to a deeper understanding of customer behavior, market dynamics, and operational efficiency. By leveraging these insights, companies can make strategic decisions that are backed by evidence rather than intuition.
Enhancing Customer Satisfaction
Moreover, data analysis plays a critical role in enhancing customer satisfaction and loyalty. By analyzing customer data, businesses can uncover preferences, pain points, and purchasing habits. This understanding allows them to tailor their offerings, personalize marketing efforts, and improve service delivery.
Meet Customer Expectations
For instance, retailers can analyze buying patterns to determine optimal stock levels or identify opportunities for upselling and cross-selling. As a result, organizations that prioritize data analysis are better positioned to meet customer expectations and foster long-term relationships.
Operational Efficiency
In addition to improving customer relations, data analysis also drives operational efficiency. Companies can scrutinize their internal processes to pinpoint inefficiencies and bottlenecks that hinder productivity. By understanding where resources are being wasted or where delays occur, organizations can implement data-driven strategies to streamline operations. This not only reduces costs but also enhances overall performance, allowing businesses to allocate resources more effectively and respond to market changes with agility.
Types of Data Analysis Techniques
Data analysis encompasses a range of techniques, each serving a specific purpose in the quest for insights.
Descriptive Analysis
Descriptive analysis provides an overview of historical data, summarizing what has happened over a given period. This technique often employs statistical measures such as mean, median, and mode to present a clear picture of past performance.
Businesses can use descriptive analysis to gauge overall trends and assess areas that require attention, setting a foundation for deeper exploration.
Diagnostic Analysis
On the other hand, diagnostic analysis delves deeper into the data to understand why certain outcomes occurred. This technique enables businesses to identify the root causes of issues and trends by examining the relationships between different variables.
For instance, if a company notices a decline in sales, diagnostic analysis can help pinpoint whether this is due to external market conditions, internal process inefficiencies, or changes in consumer preferences.
Understanding the ‘why’ behind data trends is crucial for developing effective strategies moving forward.
Predictive analysis
Predictive analysis takes data analysis a step further by utilizing historical data to forecast future outcomes. Using statistical algorithms and machine learning techniques, businesses can identify potential trends and make informed predictions about future events.
For example, a retail company might use predictive analysis to anticipate peak shopping periods, allowing for better inventory management. By forecasting customer behavior, organizations can proactively adapt their strategies to align with anticipated changes, ensuring they remain competitive in a dynamic market.
Steps in the Data Analysis Process
The data analysis process is systematic and structured, ensuring that insights are derived logically and efficiently.
Step 1- Objectives of Analysis
The first step is to define the objectives of the analysis. This involves clarifying what questions the business aims to answer and what insights are sought. A clearly defined objective sets the direction for the analysis and ensures that all efforts are aligned with the organization’s goals.
For instance, a company might want to understand customer retention rates to improve its loyalty program, making it essential to frame specific questions around this objective.
Step-2 Gathering Relevant Data
Once the objectives are established, the next step is data collection. This phase involves gathering relevant data from various sources, which could include internal databases, surveys, customer feedback, and external market research.
It is crucial to ensure that the data collected is accurate, relevant, and comprehensive. The quality of the data directly impacts the reliability of the analysis, so businesses should invest time in curating high-quality datasets.
Step-3 Data Cleaning
After data collection, the analysis phase commences. This step involves cleaning the data to remove any inconsistencies, errors, or irrelevant information. Data cleaning is essential for ensuring that the subsequent analysis is based on reliable information.
Following this, various analytical techniques are applied to uncover insights, trends, and patterns.
Step-4 Interpreting the Results
The final step involves interpreting the results and presenting the findings in a manner that is understandable and actionable for stakeholders. Clear visualization through graphs, charts, and dashboards can significantly enhance the communication of insights.
Key Tools and Software for Data Analysis
In the realm of data analysis, the right tools and software can significantly enhance efficiency and effectiveness.
1. MS Excel
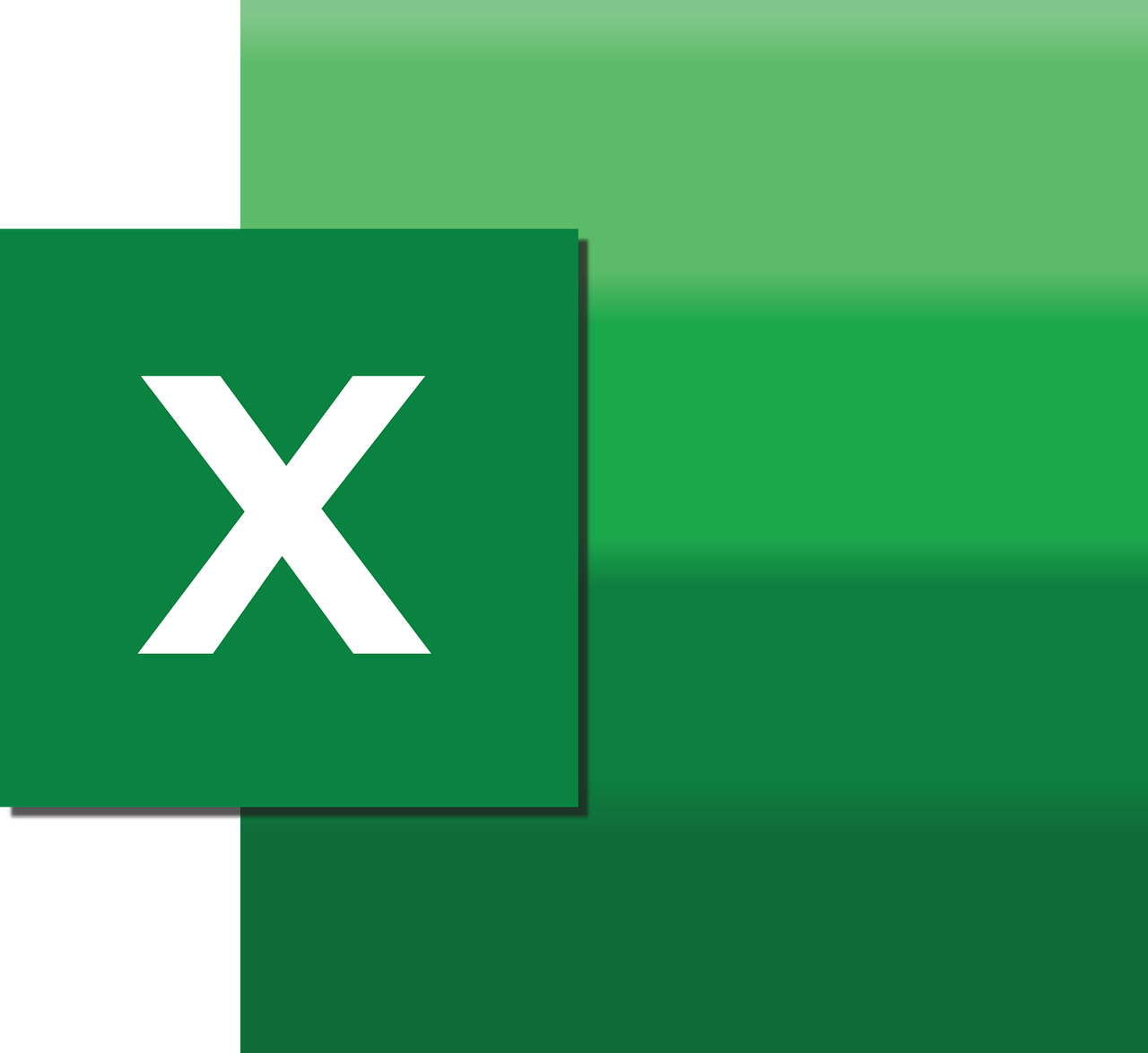
One of the most commonly used tools is Microsoft Excel, which offers robust functionalities for data manipulation, statistical analysis, and visualization. Excel’s user-friendly interface makes it accessible for users at all skill levels, while its advanced features cater to more complex analytical needs. Businesses often use Excel for initial data exploration, creating pivot tables, and generating charts to visualize trends.
1. R/Python
For more sophisticated analysis, statistical software such as R and Python has gained popularity due to their powerful capabilities and flexibility.
R for Data Analysis
R is specifically designed for statistical analysis and data visualization, making it an excellent choice for statisticians and data scientists.
Python for Data Analysis
Python, with its rich ecosystem of libraries like Pandas, NumPy, and Matplotlib, supports a broad range of data analysis tasks, from data cleaning to machine learning.
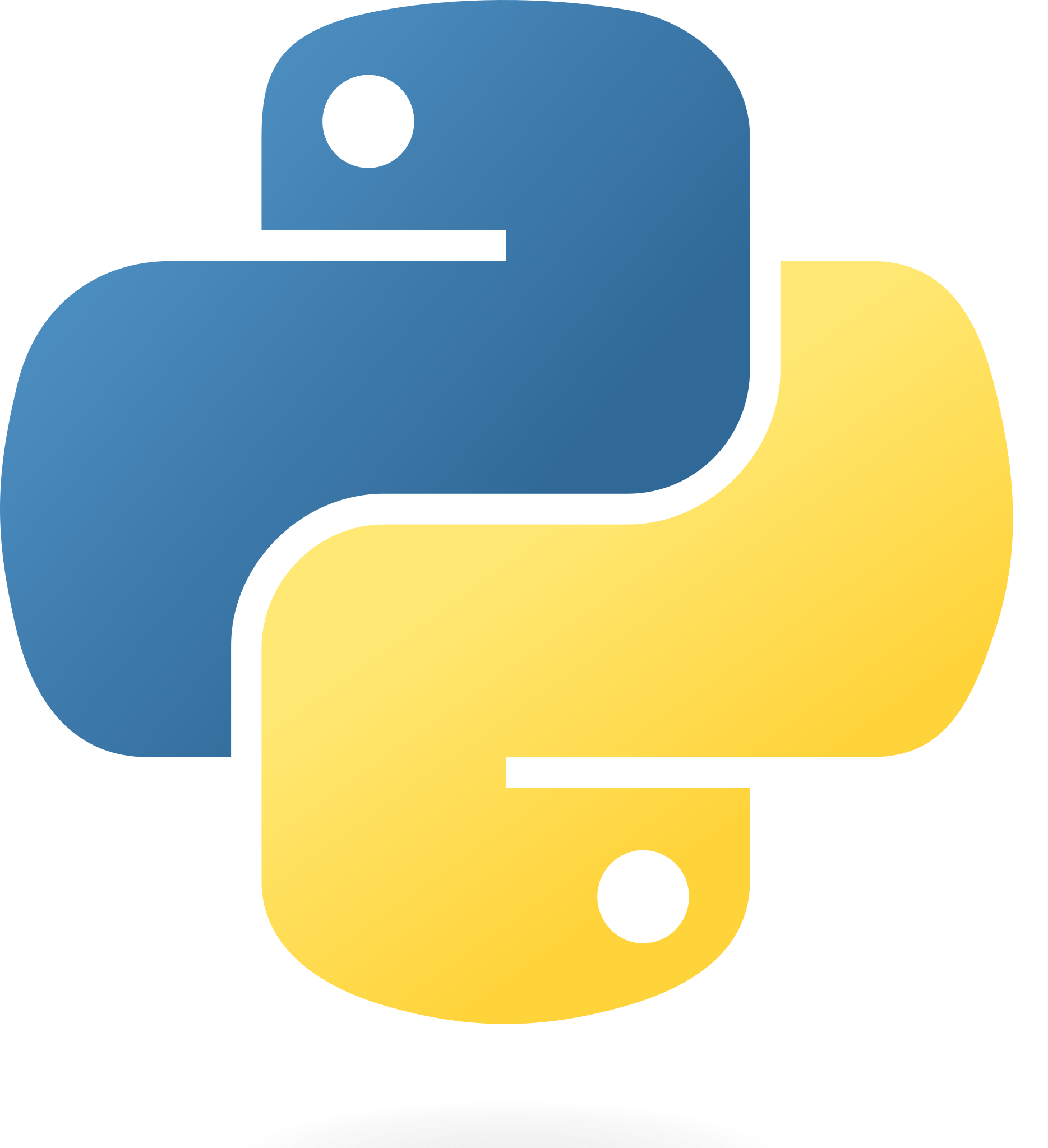
These programming languages allow users to automate processes and handle large datasets more efficiently, providing a significant advantage in today’s data-centric environment.
Business Intelligence Tools for Data Analysis
Business Intelligence (BI) tools such as Tableau, Power BI, and QlikView are also essential for organizations looking to visualize their data and generate interactive reports.
These tools enable users to create dashboards that provide real-time insights into key performance indicators (KPIs) and other critical metrics.
By simplifying the visualization process, BI tools empower decision-makers to grasp complex data quickly and make informed choices. The integration of these tools into the data analysis process can lead to more actionable insights and a stronger competitive edge.
Common Challenges in Data Analysis
1. Data Quality
Despite its many benefits, data analysis is not without its challenges. One of the primary obstacles organizations face is data quality. Inaccurate, incomplete, or outdated data can lead to misleading insights and poor decision-making. Businesses must prioritize data governance and invest in processes that ensure data integrity, including regular audits and validation checks. Without a solid foundation of reliable data, even the most sophisticated analysis techniques may yield subpar results.
2. Data Integration
Another significant challenge is the complexity of data integration. Organizations often gather data from multiple sources, including CRM systems, social media platforms, and market research reports. Integrating these diverse datasets can be a daunting task, particularly when they are stored in different formats or structures. The lack of a unified data strategy can hinder effective analysis and prevent organizations from gaining a holistic view of their operations. Companies must invest in data integration tools and strategies to streamline this process and facilitate seamless analysis.
3.Skills Gap
Lastly, the skills gap in data literacy poses a considerable challenge. As data analysis becomes increasingly vital for business success, organizations face a shortage of professionals with the necessary skills to analyze and interpret data effectively.
This gap can lead to an over-reliance on a small team of analysts, limiting the organization’s overall analytical capabilities. To overcome this challenge, businesses should invest in training programs that enhance data literacy across the organization, empowering employees at all levels to engage with data meaningfully.
Best Practices for Effective Data Analysis
To maximize the value derived from data analysis, organizations should adhere to several best practices.
1. Setting Clear Objectives
First and foremost, setting clear objectives is paramount. Before embarking on any analysis project, businesses must define what they aim to achieve, ensuring that the analysis aligns with broader organizational goals.
This clarity not only streamlines the analysis process but also enhances stakeholder engagement by demonstrating the relevance of the findings.
Another best practice is to ensure data quality through rigorous data management practices.
Organizations should establish protocols for data collection, validation, and cleaning, ensuring that the data used in analysis is accurate and reliable.
Regular audits and updates to datasets can help maintain data integrity, allowing businesses to trust their insights and decisions.
Additionally, fostering a culture of data-driven decision-making within the organization encourages employees to rely on data rather than intuition.
Moreover, effective communication of insights is crucial for ensuring that findings are actionable. Data analysts should focus on presenting results in a clear and compelling manner, utilizing visualizations and narratives that resonate with stakeholders.
Tailoring presentations to the audience’s level of data literacy can enhance understanding and facilitate discussions around the implications of the analysis.
By prioritizing clear communication, organizations can foster a culture of collaboration and informed decision-making based on data-driven insights.
Data Analysis for Decision Making and Strategy Development
Data analysis serves as a critical foundation for informed decision-making and strategic development in organizations. By providing insights into market trends, customer behavior, and operational performance, data analysis empowers leaders to make decisions that are grounded in evidence rather than speculation.
For instance, businesses can use data analysis to identify emerging market opportunities, assess competitive landscapes, and allocate resources more effectively.
With a data-driven approach, organizations can navigate uncertainty with greater confidence and agility.
Furthermore, data analysis facilitates continuous improvement by enabling organizations to measure the effectiveness of their strategies.
By tracking key performance indicators (KPIs) and analyzing outcomes, businesses can assess the impact of their initiatives and make necessary adjustments.
This iterative process ensures that organizations remain responsive to changing market conditions and customer needs, fostering a culture of innovation and adaptability.
Strategic development also benefits from scenario modeling, a technique that utilizes data analysis to explore potential future outcomes based on different variables and assumptions.
By simulating various scenarios, organizations can assess the potential impact of their decisions and develop contingency plans.
This proactive approach to decision-making equips businesses to navigate uncertainties and capitalize on opportunities as they arise, ultimately driving long-term success.
Conclusion: Harnessing the Power of Data Analysis for Business Success
In conclusion, data analysis is a powerful tool that can unlock valuable insights and drive success in today’s competitive business landscape. By understanding its importance, leveraging diverse analytical techniques, and following a structured process, organizations can transform raw data into actionable intelligence. Investing in the right tools and fostering a culture of data literacy further enhances the effectiveness of data analysis, enabling businesses to make informed decisions and optimize their operations.
As demonstrated by successful case studies across various industries, the impact of data analysis on strategic decision-making and customer engagement is profound. Organizations that proactively embrace data analysis position themselves to respond to market changes, enhance customer satisfaction, and achieve sustainable growth. In a world where data is abundant, the ability to harness its power will ultimately determine the success of businesses in the years to come.
By committing to a data-driven approach and continually refining their analytical capabilities, organizations can unlock the full potential of data analysis, paving the way for innovation, efficiency, and long-term success. As we move further into the digital age, the insights derived from data analysis will play an increasingly pivotal role in shaping the future of business.